Within the domain of Optimizely Web Experimentation Metrics, the emphasis is on objective key performance indicators (KPIs) selected to assess an experiment’s outcomes and impact. These metrics serve as essential indicators guiding experiments towards the attainment of an organisation’s overarching objectives. In this exploration, we delve into the role and significance of metrics, uncovering their influence on user experiences, conversions, and business advancement.
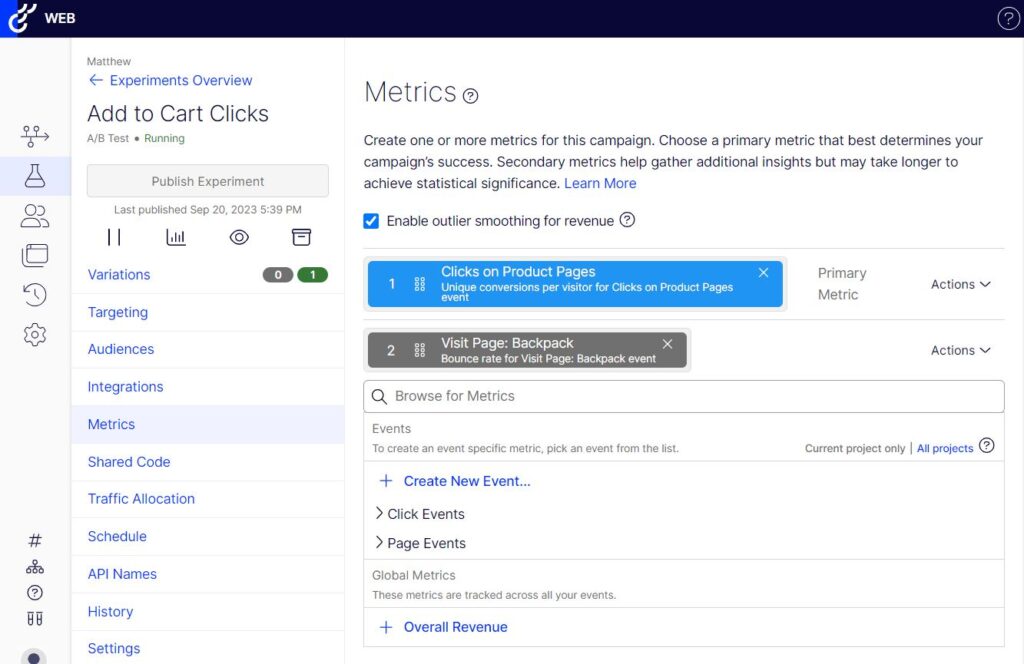
There are various types of metrics used within Optimizely Web Experimentation, these include:
Value Metrics: Value Metrics are crucial KPIs in Optimizely Web Experimentation, directly tied to organisational objectives. They include metrics like conversion rates, add-to-cart rates, bounce rates, and form completions, measuring the primary impact of experiments.
Program Metrics: Program Metrics track the overall success of experimentation initiatives. They include experiment frequency, duration, development time, idea generation, and metrics per experiment, providing insights into the experimentation program’s efficiency.
Output Metrics: Output Metrics are ultimate success measures, directly aligned with product or experience goals. Examples include account sign-ups, purchases, lead generation, or conversion rates, representing the desired outcomes.
Input Metrics: Input Metrics serve as leading indicators, reflecting user actions before reaching Output Metrics. These metrics offer insights into user behaviour patterns and may include cart page views, form field entry rates, or product page views.
Primary Metrics: Primary Metrics capture the direct user action being assessed in an experiment. They provide a clear focus on the primary objective and guide the experiment’s success evaluation.
Secondary Metrics: Secondary Metrics measure subsequent actions influenced by the primary change in an experiment. They offer a more comprehensive view of user behaviour beyond the primary action.
Monitor Metrics: Monitor Metrics, introduced later in experiments, help identify any unintended consequences of changes on visitor actions or behaviours. They provide a safety net to detect unexpected outcomes.
Value Metrics in Optimizely Web Experimentation
In Optimizely Web Experimentation, “Value Metrics” are specific KPIs or metrics that are chosen to evaluate the success and impact of an experiment. These metrics are essential because they directly tie experiment results to the organisation’s overall goals and objectives. Value Metrics are typically the most critical metrics that align with the desired outcomes of the experimentation effort.
For example, if an e-commerce website is running an experiment to optimise product page layouts, the Value Metrics might include:
- Conversion Rate: The percentage of visitors who make a purchase after viewing a product page.
- Add-to-Cart Rate: The percentage of visitors who add a product to their cart after viewing a product page.
- Bounce Rate: The percentage of visitors who leave the site immediately after viewing a product page, which may indicate a lack of engagement.
- Form Completions: Number or percentage of users who successfully submit a form. Form completions are valuable for lead generation, sign-ups, or any other actions that require users to submit information.
By focusing on these Value Metrics, organisations can assess whether the experiment had a positive impact on their core objectives, such as increasing sales or improving user engagement. It helps in making informed decisions about which variations or changes to implement permanently and which to iterate on for further optimisation. Ultimately, Value Metrics are central to the data-driven decision-making process in Optimizely Web Experimentation.
Program Metrics in Optimizely Web Experimentation
Program Metrics within Optimizely Web Experimentation refer to the KPIs and data points that are tracked and analysed to measure the overall impact and success of an experimentation program. These metrics provide insights into how experimentation initiatives are contributing to the organisation’s goals and objectives.
Program Metrics typically include:
Experiments per Month: This metric measures velocity (the frequency and volume of experiments being conducted within the organisation). It reflects the organisation’s commitment to a culture of continuous experimentation and innovation.
Duration of Experiments: This metric tracks how long each experiment runs from start to finish. It helps assess the efficiency of the experimentation process and whether experiments are being concluded in a timely manner.
Dev Time per Experiment: This metric quantifies the amount of development or engineering time dedicated to each experiment. It helps organisations understand the resource allocation required for their experimentation efforts.
Ideas Generated: The number of experiment ideas generated within a specific period indicates the organisation’s creative thinking and ideation capabilities. A higher number can suggest a vibrant experimentation culture.
Metrics per Experiment: This metric assesses the number and relevance of key performance indicators (KPIs) and metrics tracked for each experiment. It ensures that experiments are aligned with meaningful business objectives.
By tracking these Program Metrics, organisations can evaluate the overall success of their experimentation initiatives, make data-driven decisions, and continually refine their strategies to achieve their business objectives.
Output Metrics in Optimizely Web Experimentation
In Optimizely Web Experimentation, “Output Metrics” are the ultimate metrics that represent the primary goals or key performance indicators (KPIs) for your product or user experience. These metrics are the critical measures of success that directly align with the overall objectives of your experiment. Output Metrics focus on the desired outcomes that you aim to achieve with your product or experience. Some examples of Output Metrics include:
- Account Registrations: If the primary goal of your product or webpage is to acquire new users or customers, the number of account sign-ups would be a prominent Output Metric.
- Purchases: For e-commerce websites or platforms, the number of successful purchases or transactions is a fundamental Output Metric, as it directly correlates with revenue generation.
- Lead Generation: In B2B or service-oriented contexts, generating leads through form submissions or inquiries may be a critical Output Metric, as it represents potential business opportunities.
- Conversion Rates: While not a single metric, the overall conversion rate of visitors to customers or subscribers can be an Output Metric, as it encapsulates the success of your conversion funnel.
Output Metrics serve as the ultimate measure of success for an experiment. They indicate whether the changes implemented through the experiment have a meaningful impact on achieving the organisation’s broader objectives. By monitoring these Output Metrics, organisations can make informed decisions about whether to implement or iterate on the changes, as well as assess the overall effectiveness of their optimisation efforts.
Input Metrics in Optimizely Web Experimentation
In Optimizely Web Experimentation, “Input Metrics” are specific user actions or behaviours that serve as leading indicators of success and are closely related to the ultimate Output Metrics or primary goals of an experiment. Input Metrics help in understanding how changes introduced through an experiment influence user engagement and behaviour. Here are some key features and examples of Input Metrics:
- Leading Indicators of Success: Input Metrics are considered leading indicators because they precede or are strongly correlated with the ultimate success or Output Metrics. They provide insights into whether users are moving in the desired direction before they actually reach the final conversion or goal.
- Examples of Input Metrics:
- Cart Page Views: For an e-commerce website, the number of users viewing their shopping cart is an Input Metric that indicates an intention to make a purchase. It’s a step that often precedes the actual purchase (the Output Metric).
- Form Field Entry Rate: If the goal is to capture leads through a contact form, the rate at which users start entering information into the form fields can serve as an Input Metric. It indicates user engagement with the form, which is a precursor to form submissions (the Output Metric).
- Product Page Views: In the context of an online store, the number of users viewing specific product pages can be an Input Metric. It suggests interest in the product, which can lead to potential purchases (the Output Metric).
- User Behaviour Insights: Input Metrics provide valuable insights into user behaviour and interactions with a webpage or product. They help experimenters understand which specific actions users take before reaching the desired conversion point.
- Optimisation Focus: Input Metrics are often used to guide experimentation efforts. If an experiment is not directly impacting the Output Metric, analysing Input Metrics can reveal whether users are engaging with the experiment’s elements and moving toward the desired outcomes.
By monitoring Input Metrics in Optimizely Web Experimentation, organisations can gain insights into user behaviour patterns, optimise the user journey, and make informed decisions about experiment variations to ultimately drive the desired Output Metrics and achieve their primary goals.
Primary Metrics
In Optimizely Web Experimentation, the concept of “Primary Metrics” is crucial for defining and measuring the primary goal or desired outcome of an experiment. Here are the key features of Primary Metrics:
- Capture Direct User Action: The Primary Metric is specifically chosen to capture a direct user action or behaviour on a webpage that aligns with the experiment’s primary goal. This could include actions like button clicks, form submissions, product purchases, or any other meaningful interaction that reflects the desired user behaviour.
- Single Primary Metric: Optimizely allows for only one Primary Metric to be designated for each experiment. This restriction ensures clarity and focus on the most critical metric that represents the primary objective of the experiment.
- Impact Measurement: The Primary Metric is used to measure the impact of the experiment on the targeted webpage. It serves as the primary criterion for determining whether the experiment was successful in achieving its intended goal.
For example, if an e-commerce website is running an experiment to test a new call-to-action button’s colour and its impact on conversions, the “Click-through Rate” (CTR) of that button could be designated as the Primary Metric. This means that the experiment’s success or failure will be primarily evaluated based on changes in the CTR, as it directly reflects the desired user action (clicking the button) and measures what the experiment aims to impact (improving click-through rates).
By choosing the right Primary Metric, organisations can maintain clarity and alignment with their experimentation goals, making it easier to interpret results and make informed decisions about which variations to implement or iterate upon.
Secondary Metrics
In Optimizely Web Experimentation, “Secondary Metrics” are additional metrics that can be tracked alongside the Primary Metric to provide a more comprehensive view of an experiment’s impact on user behaviour. Here are the key features of Secondary Metrics:
- Up to Four Secondary Metrics: Optimizely allows for the inclusion of up to four Secondary Metrics in addition to the Primary Metric. These metrics are added after the Primary Metric has been designated.
- Measuring Subsequent User Actions: Secondary Metrics are used to measure user actions that occur after the primary action measured by the Primary Metric. These actions are often considered “down-funnel” actions, meaning they represent deeper engagement or conversion steps that occur as a result of the primary action.
- Examples of Secondary Metrics: Secondary Metrics could include actions such as “Purchase Completion,” “Lead Form Submission,” “Product Page Views,” or “Time Spent on Site.” These metrics help assess how the primary user action impacts subsequent behaviours and conversions.
For instance, if the Primary Metric is “Button Clicks” on a product page, a Secondary Metric like “Purchase Completion” can be used to track how many users who clicked the button subsequently completed a purchase. This allows organisations to understand not only the immediate impact of the button click but also its influence on the ultimate conversion goal.
Secondary Metrics provide a more nuanced understanding of user behaviour and the broader impact of an experiment on the user journey. By analysing both Primary and Secondary Metrics, organisations can gain insights into how changes on a webpage affect user interactions and conversions at different stages of the funnel.
Monitor Metrics
In Optimizely Web Experimentation, “Monitor Metrics” are additional metrics that can be added to an experiment after the Primary and Secondary Metrics, providing further insight into how an experiment may affect other visitor actions or user behaviours. Here are the key features of Monitor Metrics:
- Additional Metric: Monitor Metrics are introduced after the initial set of metrics, which includes the Primary Metric and up to four Secondary Metrics. If you need to track additional metrics beyond these first five, Monitor Metrics come into play.
- Identifies Changes in Visitor Actions: Monitor Metrics are used to identify any changes in visitor actions or behaviours that might result from the experiment. These metrics help assess whether the experiment has unintended consequences or detrimental effects elsewhere on the webpage or user journey.
- Measures Impact on Related Changes: Monitor Metrics focus on measuring things that are directly impacted by the primary change introduced in the experiment. This allows organisations to understand how alterations to one aspect of a webpage or user experience may ripple through to affect other related behaviours.
For example, if the Primary Metric is “Button Clicks” on a product page, and a Monitor Metric is “Exit Rate” (the rate at which users leave the page after clicking the button), it helps assess whether the button click, which is intended to drive engagement, has any negative effects, such as causing users to leave the page prematurely.
Monitor Metrics are valuable for ensuring that changes made in an experiment do not have unintended negative consequences on user behaviour or site performance. They provide a safety net to detect any unexpected outcomes and allow organisations to make informed decisions about whether to implement, iterate, or roll back changes based on comprehensive data.
In this article we have learned that the various types of metrics used within Optimizely Web Experimentation stand as beacons pointing the way towards success. These metrics are fundamental measures directing experimentation endeavours towards ultimate business goals. Choosing the right metrics empower us to make empirical, data-driven decisions, enhance user experiences, and realise meaningful outcomes inline with our organisational objectives.